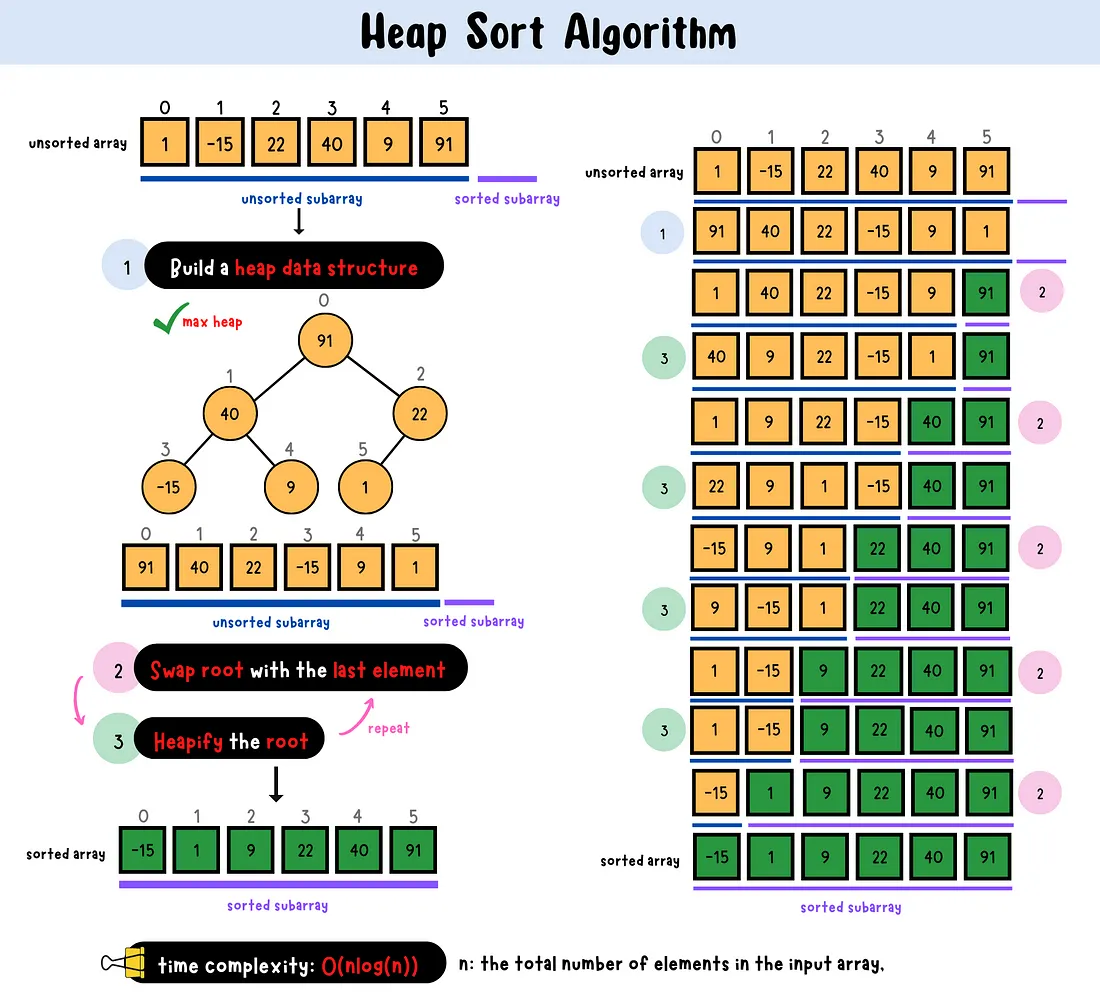
Heapsort, why it's efficient?
The heapsort algorithm was designed as a highly efficient and in-place sorting algorithm by John William Joseph Williams in the paper “Algorithm 232 - Heapsort”, published in 1964 1.
The complexity of Heapsort is
The heap data structure
Before diving into heapsort, let’s get familiar with the heap data structure.
The heap has properties that make it very suitable to build an efficient sorting algorithm.
Finding the largest element in the heap is
The heap can be visualized as a left-complete binary tree, which means all the levels of the tree are full;
they contain two child nodes, except the two last ones, which are on the bottom.
The bottom level is filled from left to right, which implies the name left-complete.
It Is precisely the left-complete property that allows the heap to be implemented using a contiguos array, without the need of using pointers, which allows heapsort to be constant
The max heap property, also known as heap order, order the nodes by
Due to the property
Heap implementation
Due to the left-complete property, we can implement a heap using a contigous array.
The (a) binary tree is represented using the (b) contiguous array 3.
The number above each node is the index of that node in the array.
We can observe that the indexes are increasing from top to bottom from left to right.
The number inside each node is the key, or the value of the node.
Given a node at index
def left(i):
return 2 * i
def right(i):
return left(i) + 1
def parent(i):
return math.floor(i/2)
Heapify
To maintain the max heap property, the heapify algorithm is applied.
Heapify receives a heap
If there is a swap, heapify executes again at the subtree of the node that was swapped. This is needed because the subtree might not be a heap due to the swap. This can happen when the value of the node is smaller than its children. We can observe this behavior in this animation made by CodesDope which ilustrates heapify 4:
def max_heapify(heap, i: int, m: int):
l = left(i)
r = right(i)
max_value = i
if l < m and heap[l] > heap[max_value]:
max_value = l
if r < m and heap[r] > heap[max_value]:
max_value = r
if max_value != i:
swap(heap, i, max_value)
max_heapify(heap, max_value, m)
Heapify runs in
To build the heap,
def build_heap(heap):
heap_size = len(heap)
n = math.floor(heap_size/2)
for i in range(n+1):
max_heapify(heap, n - i, heap_size)
Heap sorting
Intuitively, the heapsort algorithm builds the heap using
def heapsort(heap):
build_heap(heap)
heap_size = len(heap)
for i in range(heap_size):
n = heap_size - 1 - i
swap(heap, 0, n)
max_heapify(heap, 0, n)
This is an ilustrated animation of the algorithm made by CodesDope 4.
Because
Stability
Heapsort is not a stable sorting algorithm due to the
heap = [
(4, "Alice"),
(6, "Mart"),
(6, "Kage"),
(3, "Bob"),
(3, "John"),
(5, "Robert"),
(1, "Plato"),
]
When sorted using heapsort it outputs:
heapsort(heap)
# [
# 1: Plato,
# 3: John,
# 3: Bob,
# 4: Alice,
# 5: Robert,
# 6: Kage,
# 6: Mart
# ]
We can observe that the relative position of Mart and Kage as well as Bob and John are swapped. This reveals that heapsort is indeed not stable.
Conclusion
We explored heapsort an efficient, both in time
We explored the properties of the left-complete binary tree and how
I hope you have enjoyed the explanations, have a wonderful day.
Footnotes
-
J. W. J. Williams, “Algorithm 232: Heapsort”, Communications of the ACM, vol. 7, no. 6, pp. 347–348, 1964. ↩
-
CS 161 Lecture 4 - 1 Heaps. Available at https://web.stanford.edu/class/archive/cs/cs161/cs161.1168/lecture4.pdf ↩ ↩2
-
Lecture 13: HeapSort. Available at https://www.cs.umd.edu/users/meesh/cmsc351/mount/lectures/lect13-heapsort.pdf ↩ ↩2
-
Heapsort. Available at https://www.codesdope.com/course/algorithms-heapsort/ ↩ ↩2